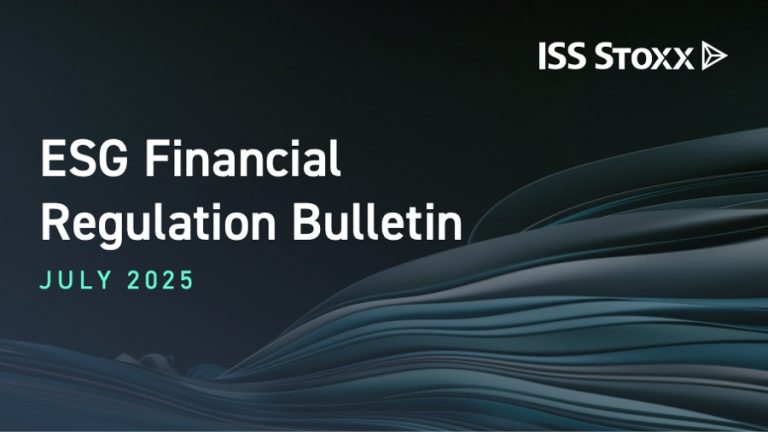
The Latest in ESG and Stewardship Regulation – July 2025
ISSB IFRS Foundation Publishes Jurisdictional Profiles Evidencing Progress Towards Adoption of International Sustainability Standards Board Standards The IFRS Foundation published on June 12 a set