Digitalization and advances in artificial intelligence (AI) have come with a loss of privacy. The collective cost of what we share, from the time we wake up and check our phones until our watch finishes tracking our sleep the following evening, exposes a market failure that privacy regulation alone cannot fix. Businesses can look to digital service taxes (DSTs) and liabilities for recent data breaches to discern the potential ‘social cost of data’ related to their activities. For investors, the ISS ESG Corporate Rating provides support in determining how well businesses are addressing privacy issues.
The Right to Privacy…
The definition of privacy has been evolving since at least the late nineteenth century, often in response to new technology. Privacy has been recognized as a fundamental human right: Article 12 of the Universal Declaration of Human Rights states “No one shall be subjected to arbitrary interference with his privacy, family, home or correspondence, nor to attacks upon his honour and reputation.”
Meanwhile, digitalization, which touches almost every aspect of modern life, combines with AI to create the capacity for unprecedented privacy loss. The UN Human Rights Council recognized these threats back in 2012, particularly in the context of surveillance. The UN Special Rapporteur on the right to freedom of opinion and expression has emphasized that the rights to privacy and freedom of expression are closely connected.
…Is Worth a LOT
Individuals clearly place a high value upon their privacy. For the largest online platforms, the annual US$ value of privacy to their users is likely in the billions or tens of billions. Privacy values for individuals have been estimated through stated preference discrete-choice experiments, including in the context of online data privacy (Prince and Wallsten [2021] and Savage and Waldman [2015]).
Privacy concerns may be increasing over time. Acquisiti et al. (2015) note a study of social media disclosures indicating less sharing of profile information with the public over time as cross-context data-sharing practices became more widely known. Also, Baye and Prince (2020) suggest that observed privacy values may be linked to the relative market power of corporate entities, such that dominant firms may impose shadow prices with respect to privacy that would be lower in a more competitive market.
Measured against the goal of maximizing social welfare, digital platforms usually collect too much data, according to a literature review on privacy-related externalities by Goldfarb and Que (2023). Even if individuals are fully informed in the exchange of their own privacy for products or services, negative externalities result. They result because information about individuals can be used to predict the preferences and behaviors of those who are in some way similar (i.e., profiling). Youyou et al. (2015) find that a few hundred Facebook likes is sufficient to judge someone’s personality better than a spouse can.
AI Has Increased Privacy Threats…
Facial recognition software can scrape billions of images from social media platforms and be sold to law enforcement and private enterprise for surveillance, and voice assistants can record and store personal conversations. Generative AI enables synthetic media, or deepfakes, that can result in non-consensual explicit content and disinformation. Sophisticated chatbots can engage in human-like conversations, all while collecting reams of personal or confidential information.
Efforts at data protection may be compromised as AI systems improve. De-identification, or the removal of personal information from a data set, may be rendered useless. Culnane et al. (2017) were able to re-identify anonymized data from an open health data set.
…And Regulation Has Limits
The UN Conference on Trade and Development’s Global Cyberlaw Tracker reported that in 2021, 137 out of 194 member countries have adopted local legislation that includes clear guidelines on the collection, use, and protection of personal data and privacy. In Europe, 98% of countries have privacy regulations, followed by the Americas at 74%, and Africa and Asia at 61% and 57%, respectively.
The EU’s General Data Protection Regulation (GDPR) is the current gold standard for privacy regulation. Businesses that offer products and services in the EU are required to meet strict data protection principles, including transparent and legitimate data collection and processing measures, data minimization and storage limitation commitments, and procedures that guarantee the accuracy and security of data held. The ISS ESG Corporate Rating evaluates adherence to the GDPR principles on a global basis.
However, privacy regulation has clear limits. Greater user consent to collection and control of data and tighter corporate security measures cannot address the privacy loss from profiling, whereby information disclosed by the crowd negatively affects the individual who reveals seemingly innocuous information. It fails to control the capture of too much data in the first place.
Targeted regulation of AI models is nascent. China and the EU have proposed frameworks that require transparency (which may aid the traceability of model decisions, even if the decisions are not fully explainable), risk assessments, oversight, and, in some cases, preclearance. Certain AI models may be prohibited – for example, the EU’s proposed AI Act would ban social scoring, i.e., evaluating the trustworthiness of people based upon their behavior across several contexts. AI model regulation may mitigate some harms, but it is too blunt of an instrument to calibrate the right level of personal data collection.
Toward a Social Cost of Data?
Public Measures
Taxes can be used to internalize externalities (e.g., excise taxes on tobacco, alcoholic beverages) when regulation falls short. Efforts to address tax shortfalls aggravated by increased digitalization of the global economy may provide an opportunity to address privacy-related externalities as well.
Several European countries have enacted digital services taxes (DSTs) in recent years to address the erosion of their tax base from internet services originating outside their borders. OECD countries are currently negotiating a treaty to revise global tax rules, which may ultimately replace many (though perhaps not all) of the DSTs. Nevertheless, the DST rates may be instructive to investors and digital businesses as examples of a politically viable measure. A typical DST in Europe is roughly 2% to 3% of internet advertising revenue. The U.S. state of Maryland has a similar levy, with rates ranging from 2.5% to 10%. Other U.S. state tax proposals may await the outcome of litigation – Maryland’s law was recently upheld by the state’s highest court.
Most DSTs are based upon revenue, but Avi-Yonah et al. (2022) argue for a volume-based tax on collected data, measured in gigabytes. This proposal aims to circumvent criticism that DSTs are income taxes in disguise. Further, the social benefit of a volume-based data tax is that it may directly and efficiently serve to mitigate the social cost of data collection.
Rubinstein (2021) makes a similar proposal from the perspective of addressing consumer exploitation, noting that privacy laws have proven ineffective and ownership approaches (whereby consumers get ‘dividends’ associated with their data) are limited in their effectiveness by intellectual property laws that do not encompass factual information. Fainmesser et al. (2022) advocate a two-pronged public policy that combines a minimal data protection requirement and a tax proportional to the data collected to achieve an optimal level of data sharing.
Private Measures
Digital businesses and their investors might benefit from proactively accounting for a social cost of data using existing DSTs as a guidepost. One approach is to anticipate the possibility that today’s DSTs migrate from a gross revenue base to a volume-of-data-collected base. Companies could calculate a cost per gigabyte by dividing their data collection volumes into 2-3% of their revenue. This social cost of data could then be built into the financial model, providing an incentive to optimize the company’s mix of data collection and data protection efforts.
A level of 2-3% of revenue is likely a small fraction of users’ privacy value and is not unreasonable as a measure of the externality: US$2 per month per application is a conservative estimate of the value of consumer privacy. Applying this to large digital businesses that disclose their user base, user privacy value equates to at least 30-40% of the annual revenue of the typical advertising-driven business.
Users may be compensated for giving up their data. The only cause for concern is the privacy loss that is uncompensated, either because consumers are ignorant of the extent and effectiveness of data collection, or because AI-enabled profiling can leverage the minimal data that the user bargains away.
Another approach to modeling the social cost of data is to use empirical evidence of liabilities stemming from corporate data breaches or other privacy violations. Most legislation limits fines to 4-6% of one year’s revenue for serious violations, though the very largest liabilities have exceeded that (Figure 1).
Figure 1: Privacy-Related Liabilities (> US$100 Million), as Percentage of Annual Revenue
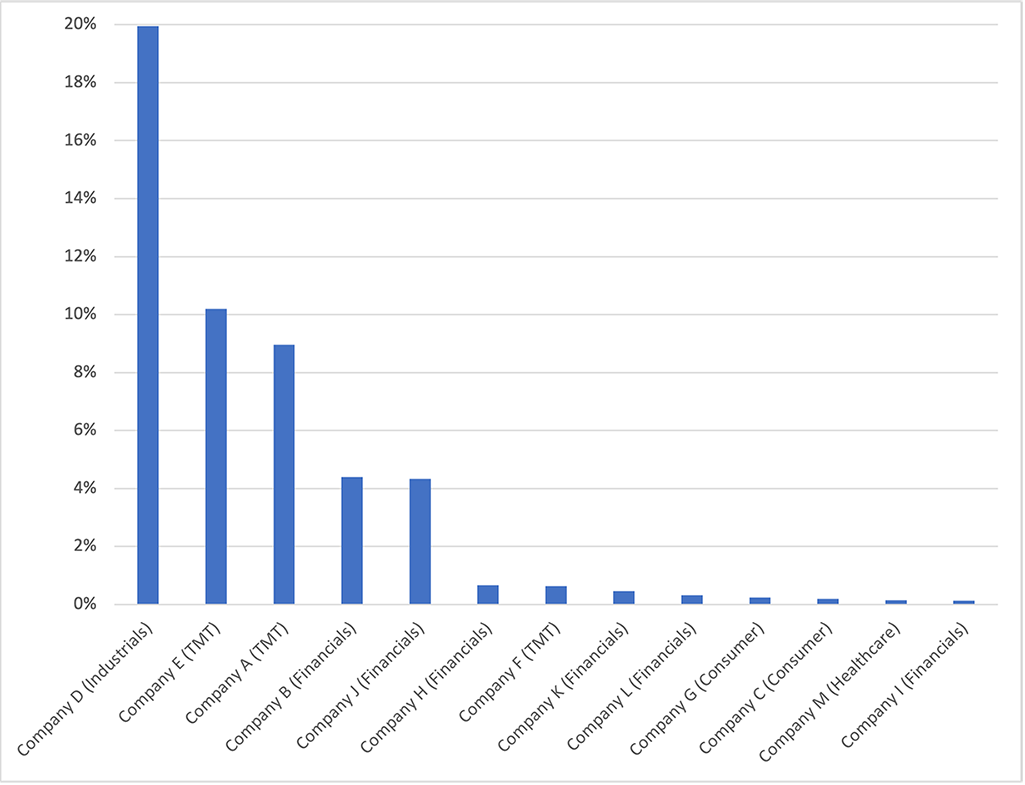
Note: TMT = Technology, Media & Telecommunications.
Source: Enzuzo.com, company public filings, and ISS ESG estimates
AI systems have varying degrees of risks. Companies and investors may be able to use a classification system to further calibrate the ranges of their social cost-of-data estimates. The AI Act classifies applications into four risk categories: unacceptable risk (e.g., social scoring, mass surveillance), high risk (e.g., recruitment, medical devices, law enforcement), limited risk (e.g., chatbots), and minimal risk (e.g., video games).
Mitigating AI-Related Privacy Issues
Once investors have a sense of the potential social cost of data, they can assess how well each company addresses it. Transparency, risk assessments, user control, and data protection are four key pillars of mitigating a company’s potential privacy liabilities. The ISS ESG Corporate Rating enables investors to evaluate progress in each area to help determine the level of unmanaged privacy risk in their portfolios.
Explore ISS ESG solutions mentioned in this report:
- Identify ESG risks and seize investment opportunities with the ISS ESG Corporate Rating.
Authored by:
Joe Arns, CFA, Sector Head, Technology, Media & Telecom, ESG Corporate Ratings
Harriet Fünning, Associate, ESG Corporate Ratings
Gayathri Hari, Analyst, ESG Corporate Ratings
Rachelle Piczon, Associate, ESG Corporate Ratings
Iona Sugihara, Associate, ESG Corporate Ratings