Recent advances in Artificial Intelligence (AI) have ignited both hopes and fears about the technology, and attitudes about AI’s potential impact on physical safety epitomize these polarized perspectives. Optimists see a newfound ability to mine data both to improve health and to remove humans from dangerous work. Pessimists fear an apocalypse brought about by machines that disregard societal values, including that of human life. Reality, at least in the nearer term, appears likely to land far from the extremes.
The maturation of AI models in the cyber-physical realm will undoubtedly entail risks for developers and users. The largest financial risks for companies may be in mass consumer markets, where, as machines become more autonomous, the law allocates an increasing share of liability for AI’s harms to its developers. Rather than guarding against a potential ‘black swan’ AI event, investors might instead delineate expected financial costs and risks related to safety impacts across their portfolio as AI develops. The ISS ESG Corporate Rating can help them make these assessments.
AI Both Offers Safety Improvement Opportunities and Poses Safety Threats
AI may accelerate the achievement of the UN Sustainable Development Goals, particularly Goal 3: Good Health and Well-Being. Natural language processing tools can convert electronic health records into analyzable information, while clinical decision support systems can leverage historical and patient data sets, and robot-assisted surgery can be used for greater precision. Food safety may also be improved with AI systems, through means such as supply chain optimization and detection of foodborne pathogens.
In the industrial sector, AI’s safety use includes monitoring, such as screening for personal protective equipment compliance in designated areas, detecting hazards and spills, and analyzing facial expressions for signs of fatigue in workers operating heavy equipment. In transportation, AI can be used to enhance safety through autonomous driving. Automatic parking, automatic emergency braking, driver drowsiness detection systems, and blind-spot monitoring can significantly increase road safety. Similarly, the technology can be used for efficiency and safety in maritime and aviation transport.
However, when physical safety depends on AI, the reliability of AI systems takes on heightened importance. System reliability and fairness matter more when failure may lead to injury or death. The greatest benefits therefore may flow from AI systems optimized along the dimensions of reliability and security.
In the longer run, AI promises to improve human safety across domains such as transportation and medical care. The problem is that AI systems need to learn on the job to get there, bringing with them near- and medium-term threats to welfare from model failure.
Ronan et al. (2020) argue that fully autonomous systems are not yet reliable without human supervision, because the vulnerabilities of such systems are still largely unknown. A reliable AI system needs to demonstrate the capacity to handle unexpected situations, potential hardware failures, and fluctuations in its environmental conditions. This resilience is vital to accident prevention.
Reports of collisions caused by a self-driving vehicle and chatbots that dispense dangerous health advice are just a few examples of AI’s immaturity. Amodei et al. (2016) define AI accidents as unintended and harmful behavior that may emerge from poor design. According to Yampolskiy (2016), these accidents may be caused by mistakes during the learning phase of AI development (e.g., faulty objective functions) or during the performance phase (e.g., vulnerability to novel environments).
Information security is critical. AI systems therefore need to withstand adversarial attacks to safeguard privacy and safety, especially in areas such as healthcare, autonomous vehicles, and utility infrastructure. Robust systems need to adequately defend against unauthorized access to intellectual assets and malicious manipulation.
The Center for AI Safety highlights how the malicious development and use of powerful adversarial AI could cause widespread harm without proper management. For instance, powerful AI systems potentially could be used to create and weaponize novel pathogens.
Military applications of AI technology present a unique security challenge. Numerous lives may be saved by using AI robots for landmine removal and identifying and safeguarding non-combatants. However, lethal autonomous weapons systems create a global security risk if compromised by adversaries. The United Nations suggests that the rise of such systems could lead to an arms race between competing nations if international cooperation on their use is not agreed upon.
AI-Related Liability Is Evolving
Civil law has only begun to wrestle with the question of who is liable for physical harm that can be traced back to AI accidents. As such, companies in an AI-infused value chain, from software providers to cyber-physical system builders (e.g., advanced driver assistance systems) to operators (e.g., robotaxi services) may be subject to risks that are difficult to quantify.
Historically, operators have borne the brunt of responsibility for accidents related to automation. Guerra et al. (2021) note that airlines, not the manufacturers, have been held liable for autopilot system failures. This is largely because of the duty of pilots to monitor and override automated features if they are deemed unsafe.
A similar trend towards holding manufacturers responsible can be seen in robot-assisted surgery. Intuitive Surgical, Inc., a large provider of robotic systems for minimally invasive surgical procedures, faced a wave of lawsuits after more than a decade in business. Yet the settlement of product liability claims has exceeded 5% of annual gross profit only once (Figure 1) in the company’s history.
Figure 1: Intuitive Surgical: Product Liability as % of Gross Profit
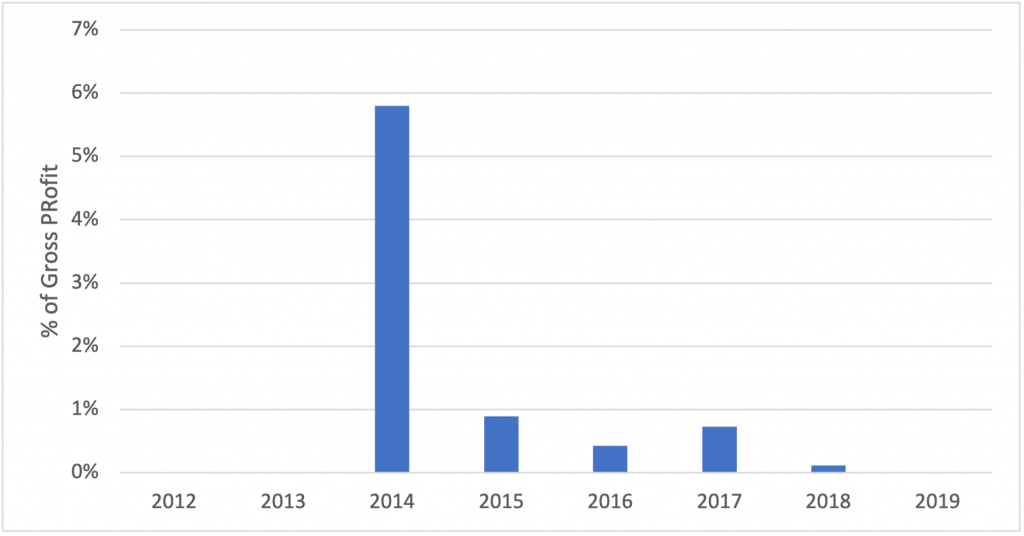
Source: Company public filings, ISS ESG
The liability question gets trickier the further removed a human agent is from the machine. An electric vehicle manufacturer can use disclaimers and warnings that keep safety responsibility in the laps of drivers for most current systems, but this may be effective only for cars with limited self-driving capabilities.
The Society of Automotive Engineers identifies six levels of driving automation, with machines assuming greater levels of responsibility at higher levels. Today, most private vehicles with automated driving features are classified at level 2 or below, which requires human monitoring at all times. Vehicles with level-3 systems have been brought to market this year, but these still require a person to be ready to assume control of the vehicle with a warning. Level-4 systems designed without the need for human attention at all are also on the road, albeit with additional restrictions (these are only approved for commercial driverless taxi services as of this writing).
Robertson (2023) argues that liability must be fairly allocated between humans and machines as driving becomes collaborative. More generally, Guerra et al. (2021) support blending negligence-based rules and strict manufacturer liability features for autonomous machines. The recommendation is to hold operators and victims responsible for harms due to their negligence, with the residual liability flowing to the manufacturer.
If this legal shift comes to pass in major geographic markets, the consequences for those in the autonomous machine value chain could be significant. System manufacturers could potentially face much greater liability than in past eras of automation, in which one or more individuals exerted some level of control over the machine.
The potential risk varies by domain. Industrial operations, such as manufacturing plants and warehouses, likely will have more limited risks from harms caused by advanced robots. In the U.S., industrial robots accounted for only 41 workplace deaths in the 26 years ending in 2017.
Industrial environments can be highly controlled, reducing the number of variables that machines need to master to ensure worker safety. In addition, employees generally cannot seek injury claims against their employers if they are covered by a workers’ compensation scheme. This circumscribes corporate liability for workplace accidents.
Medical robots may carry increased liability risk as they become more autonomous, but such a development may be tempered by the need for regulatory approvals before taking new products and devices to market. Nevertheless, the potential life-altering potential of AI in drug discovery, medication management, implantable devices, and hospital equipment is not without downside risks for providers.
The biggest risks from AI-enabled automation appear to be in mass consumer markets such as automobiles. The annual economic cost of vehicle crashes in the U.S. alone is estimated to exceed $300 billion. Even with additional regulation, a shift in liability towards manufacturers and away from consumers may merit investor attention.
Surveillance and Risk Control Efforts Can Mitigate Safety Impacts
Wang et al. (2020) suggest the implementation of a responsible AI program can be grouped into four main practices: data governance, ethically designed solutions, human-centric surveillance and risk control, and training and education. Surveillance and risk control are the most pertinent in addressing the reliability and security dimensions of AI models.
Data related to impact-mitigating product safety practices can be combined with risk exposure analysis to provide a more comprehensive view of investor risk. Those concerned with AI’s impact on safety might benefit from focusing their risk analysis upon mass market goods and services companies, such as those in the consumer discretionary and health care sectors (and the upstream suppliers that serve these end markets). Also, it may be important to differentiate risk by geography, as the legal liability standards for AI-related safety harms may evolve at varying speeds and along divergent paths.
The ISS ESG Corporate Rating already offers several indicators that address product reliability and security in a general sense, including offerings infused with AI. For instance, measures to ensure customer security assess software companies based upon the level of attention given to software development practices such as code reviews, developer-specific training, and various technical safeguards (e.g., malware detection, intrusion prevention, firewalls). Our product safety topic separately addresses components of the product value chain, from product design and development to production processes through to customer support and protection. Companies scoring higher on these metrics may be more likely to mitigate the potential safety impacts of their offerings.
Explore ISS ESG solutions mentioned in this report:
- Identify ESG risks and seize investment opportunities with the ISS ESG Corporate Rating.
By: Joe Arns, CFA, Sector Head, Technology, Media & Telecom, ESG Corporate Ratings
Ishani Deshpande, ESG Corporate Ratings Analyst
Gayathri Hari, ESG Corporate Ratings Analyst
Nat Latoza, ESG Corporate Ratings Analyst
Iona Sugihara, ESG Corporate Ratings Specialist