The release of generative Artificial Intelligence (AI) platforms to the broader public (e.g., ChatGPT/ChatGPT Plus, DALL-E 2, Stable Diffusion, Bard, GitHub Copilot) has recently intensified the debate around the potential harms of AI. When ChatGPT and Bard are prompted to return the ‘top potential harms of AI,’ bias and the related harm of discrimination takes the number two spot on the list, behind only job displacement.
Powerful generative AI that can create fake photos of fashion-forward religious figures is new, but less flashy AI has been transforming software for a generation. In the domain of hiring, where bias and discrimination are perhaps most impactful, commercialized software infused with AI has been used since the early 2000s and has provided researchers with a rich vein of data to explore the technology’s impact on fairness.
The balance of evidence suggests that AI can help companies combat discrimination in hiring practices, provided the algorithms are fairness-aware and the humans involved trust the model. The presence of AI-assisted hiring practices may provide investors with insight into which portfolio companies are likely to improve their diversity metrics and reduce discrimination-related liability.
Bias and Discrimination in AI
Bias and discrimination are often used interchangeably, but they are distinct in the context of AI models. Bias refers to systematic errors that cause results to deviate from the true relationship between the variables being modeled. Discrimination is unequal treatment due to gender, race, or other attributes that results in allocative harm, such as the denial of equal opportunity to jobs or access to credit. AI models risk entrenching existing discrimination in human-driven decisions as well as creating such discrimination due to the unintentional presence of bias.
There are two primary sources of AI model bias: biased training data, and biased developers and users. Both are reflective of societal imbalances past and present.
Any training data that is unrepresentative of the target population is an obvious source of bias. If a text-to-image model is trained only on pictures of male CEOs, the output from prompts such as “What does a CEO look like?” won’t reflect reality. In some cases, data bias may be more difficult to detect. Proxy bias results when seemingly neutral characteristics are highly correlated with more sensitive ones, such as a predictive policing algorithm using ZIP codes to predict crime incidence in highly segregated cities that effectively substitutes the neutral attribute of geography for racial mix. Even if training data is representative, algorithmic bias can result if the design of the model results in patterns that correlate with sensitive features such as gender or race. For example, a review of one large social media ad platform suggests it served job ads for certain professions based upon gender.
The creators of the AI model are another source of bias. According to Stanford University’s latest Artificial Intelligence Index Report, nearly 80% of new AI PhDs were male in 2021 and the percentage of female AI PhDs had increased by only 3 percentage points from 2011. If development teams are not representative of the populations their products serve, there is a greater likelihood that cultural biases are reflected in the model as the diversity of viewpoints may be constrained. Such teams may fail to recognize and correct potential biases in the AI models they construct. In addition to algorithmic bias, there may be labeling bias if data is subjectively assigned to certain categories.
Biases can also emerge in AI systems that learn and modify based upon human interactions. If biased information is present in user feedback, the system may reinforce and maintain those biases over time. Whittaker et al. (2021) find that some content recommendation engines amplify extreme content based upon user engagement patterns. Some AI chatbots have been tricked into spewing misinformation and hate speech mere days after being released to the public.
The Path to AI Model Fairness
A primary way to improve AI model fairness is the specification of fairness-aware algorithms. This means that in addition to other objectives, such as predicting high job performance, user engagement, or other successful outcomes, the model also factors in fairness metrics such as gender balance. These constraints encourage predictions that are equitable across certain protected attributes, thereby mitigating discrimination.
In addition to adjusting the algorithm, training data may be augmented or selected using stratified sampling. The model may also be trained with adversarial data designed to expose bias to ensure the model can withstand it. Several open-source toolkits, including IBM’s AI Fairness 360, Microsoft’s Fairlearn, and Alphabet’s What-If are available to help development teams detect and remove bias from their models. Certain evaluation tools offer the ability to continually monitor the model’s fairness over time.
Achieving fairness can involve trade-offs. Mitigating bias against one demographic group may inadvertently aggravate it against another. For example, Nugent and Scott-Parker (2022) note the potential for AI systems to discriminate against people with disabilities. Measures to ensure fairness may also introduce complexity, making it more difficult to explain model results. Such lack of interpretability hampers trust and may prevent wider adoption. Fairness may also run into regulatory hurdles. Hunkenschroer and Luetge (2022) note that the EU’s General Data Protection Regulation provides a right to explanation about algorithmic decisions. Elimination of all bias to avoid discrimination may also sacrifice accuracy with respect to the primary objective.
Fairness is context dependent and can be subjective, which makes it even more difficult to achieve the appropriate balance between performance and bias control. The same AI model may be fair for one use and unfair for others. A model that returns an image of a room filled with 100 CEOs might generate pictures that are skewed toward males. If the purpose of the model is to describe what a room of CEOs can be expected to look like, the model might be fair, and any adjustments would reduce accuracy. But if the purpose is to model what the room should look like, it would be clearly discriminatory.
The Effects of AI in Hiring
AI systems have long been in use across several stages of new employee hiring, including drafting of job descriptions, screening of candidate profiles, cognitive and behavioral trait assessment, and analysis of interview responses. Textio and TalVista are examples of tools that analyze the job descriptions provided by employers, and assesses the language used to identify potential biases or discriminatory language that could deter candidates. Johnson & Johnson, Cisco Systems, and McDonalds are among the companies utilizing these tools. Affinda and job posting platforms like Microsoft’sLinkedIn are examples of software that parse candidate profiles for job fit.
Pymetrics illustrates the use of AI and neuroscience-based games to evaluate candidates. Such platforms aim to eliminate demographic and background information from the process, focusing solely upon cognitive and behavioral traits to assess candidate suitability. Users of psychometric tests include Boston Consulting Group, JP Morgan,and Colgate Palmolive. HireVue is an example of an AI system whose online video interviewing platform analyzes candidate responses, considering factors such as language, facial expressions, tone of voice, and other non-verbal cues. It can also assess the content and structure of the answers. Unileverand Mercedes Benz have been known to use such software.
Do AI tools affect hiring discrimination? A high-profile 2018 news story reported on a large company that had reportedly developed an AI recruiting tool that was biased against women. Due to these biases, use of the algorithm was apparently abandoned.
High-profile failures can receive outsized attention. Humans and their AI creations are both afflicted with bias. The relevant question is whether AI models can mitigate discrimination compared to humans, not whether they are perfect.
The balance of the literature suggests that AI can have a positive effect on hiring discrimination. Will et al. (2023) reviewed several studies and found that AI is mostly better than humans at improving diversity and performance—although their review also found that both recruiters and candidates have the opposite perception. The authors note that the diversity improvement depends upon the use of fairness-aware algorithms rather than static supervised learning models. For example, Datta et al. (2015) and Lambrecht and Tucker (2019) note instances of job advertisements that amplify human biases. Similarly, Li et al. (2020) find that supervised learning models may entrench bias, while fairness-aware algorithms reduce discrimination.
Pisanelli (2022) indicates that among the largest companies in Europe and the US, use of AI by firms in hiring is associated with a 2% increase in the probability of hiring a female manager. Notably, use of AI is also found to be correlated with a lower probability of being sued for gender discrimination in hiring.
Technical solutions to ensure fairness-aware AI in hiring are necessary, but potentially insufficient to spur full adoption. Widespread acceptance of hiring AI may only come with successful outcomes and the passage of time. The perception that decisions made by algorithms are less fair and less trustworthy than decisions by a human is known as “algorithm aversion” according to Mahmud et al. (2022). Fleck et al. (2022) suggest that even if reduced hiring discrimination can be demonstrated, the lack of perceived fairness could be an impediment unless there is improved transparency and use of explainable models. However, Hofenditz et al. (2022) find that even explainable AI may be ineffective in altering human reactions to model recommendations.
Finally, while Hunkenshroer and Kriebitz (2023) assert that AI does not inherently conflict with human rights in a recruiting context, they highlight that factors such as the potential cost of privacy must be considered as well. For example, video tools could identify health conditions or other information that job candidates would not otherwise consent to disclose. Depending upon the tool and use case, employers may need to balance privacy concerns against potential diversity benefits when considering adoption.
Hiring AI May Be an Important Action to Promote Workplace Diversity
Measures to Promote Equal Opportunities and Diversity is an ISS ESG Corporate Rating indicator that evaluates a company’s assignment of responsibility, targets, actions and programs, grievance procedures, and audits and evaluations with respect to diversity and non-discrimination. The use of hiring AI is treated as a positive action step that companies may take as part of an overall approach to diversity. Technology-related companies might be expected to be among the early adopters of hiring AI since they are developing such tools. Our data suggests that many technology companies have steadily improved their diversity measures over the past few years, with larger companies putting in place the most robust programs.
Figure 1: Measures to Promote Equal Opportunities and Diversity: Numerical Scores for TMT Companies
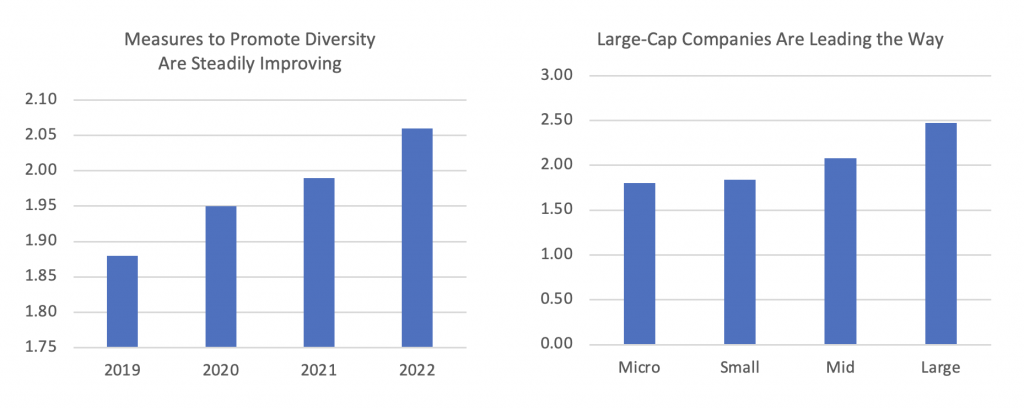
Note: TMT (Technology, media, and telecom) industries. The indicator evaluates the existence and quality of the company’s measures with regard to equal opportunities and diversity and inclusion. To achieve the maximum grade, the company needs to implement comprehensive measures to promote equal opportunities and diversity, including communication of the non-discrimination principles to its employees, clear assignment of responsibilities, strategic targets, action plans and/or programs, trainings, grievance procedures, and audits and evaluations. The measures may not be restricted to only one target group.
Source: ISS ESG
Adoption of hiring AI is likely to be a slow process that builds across the recruitment steps, from screening and ranking profiles to making hiring decisions. Explainable or more transparent AI likely cannot substitute for investing time in building trust with employers and candidates, despite evidence that it can help companies combat discrimination. Nevertheless, investors engaging with companies may wish to highlight the potential diversity benefits of AI, and to factor corporate use of hiring AI into their own ESG evaluations. The ISS ESG Corporate Rating can support investors as they consider AI’s role in promoting diversity and non-discrimination.
Explore ISS ESG solutions mentioned in this report:
- Identify ESG risks and seize investment opportunities with the ISS ESG Corporate Rating.
Authored by:
Joe Arns, CFA, Sector Head of Technology, Media, and Telecom, ESG Corporate Ratings
Hittarth Raval, Analyst, ESG Corporate Ratings
Archana Shanbhag, Analyst, ESG Corporate Ratings
Roman Sokolov, Analyst, ESG Corporate Ratings
Iona Sugihara, Associate, ESG Corporate Ratings